Research Spotlight:
Leyla Isik
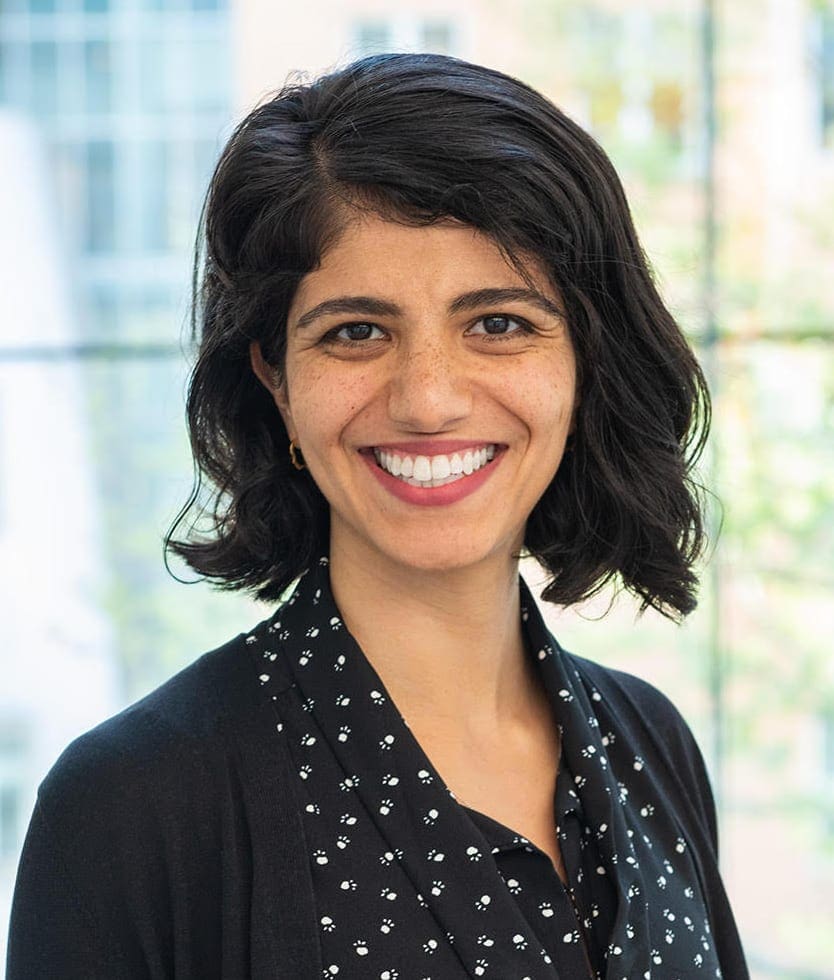
Dr. Leyla Isik is the Clare Boothe Luce Assistant Professor of Cognitive Science at Johns Hopkins University.
Her research focuses on understanding the cognitive and neural basis of human vision and social perception, and how we might implement these abilities in AI systems.
Q: What is the focus of your research?
LI: “My research centers on human vision and social perception, particularly how we quickly and effortlessly recognize complex information about other people. I investigate the neural computations that support these abilities.”
Q: What initially sparked your interest in studying how humans rapidly process complex visual and social information?
LI: “My interest in vision began with understanding the challenge it posed for computers. I was fascinated by how our brains effortlessly solve the complex problem of object recognition. We interpret a flood of visual data, identifying objects and people without conscious effort. This led me to social perception, another area where humans excel with ease but where there remains a significant gap between human and AI capabilities.”
Q: What aspects of human action and social interaction recognition are you focusing on? Are there specific social scenarios or visual contexts that you are studying to understand these processes better?
LI: “We approach this from several angles. Our research combines behavioral studies, computational modeling, cognitive neuroscience, and neuroimaging. For example, we use fMRI to study how people respond to various stimuli, ranging from simple motion capture videos that represent human forms with minimal visual details, to classic animations like those by Heider and Simmel, which depict social interactions with simple shapes. We also use more complex stimuli, such as old television shows, to explore how people integrate visual and linguistic information in real-world scenarios.”
Q: How do you utilize human neuroimaging and intracranial recordings to understand human visual and social information processing?
LI: “We do it in a few different ways. We employ neuroimaging data to correlate brain activity with behavioral responses. For example, if we have behavioral judgments about whether a scene depicts communication or conflict, we analyze which brain regions and neural patterns correspond to these judgments. We also compare brain data with computational models, both those developed in our lab and existing deep learning algorithms, to understand the similarities between these models’ internal representations and human brain activity.”
Q: How do you integrate machine learning/artificial intelligence with neuroimaging and behavioral data to uncover insights about human perception?
LI: “Sometimes we process the same stimuli shown to participants in fMRI scanners through machine learning models. This allows us to compare how computer vision models and human brains process static and dynamic scenes. While we’ve found similarities in processing static images, discrepancies in dynamic social contexts suggest there are unique aspects of human perception that current AI systems do not fully replicate.”
Q: How has computing impacted how we approach the study of visual and social information processing?
LI: “Computing has been crucial in our research. For instance, analyzing responses from 350 current AI models and comparing those to brain data is a hugely computationally intensive task that would not have been possible without our current computing resources. Similarly, analyzing fMRI data from full 45-minute movies involves handling pictures of someone’s brain every one and a half seconds, which would be challenging without advanced computing tools.”
Q: What are the next steps or future directions for your research?
LI: “One area that we have started exploring is how social perception and visual processing change, both in typical and atypical development. We analyze publicly available data from three-year-olds and adults watching the same movie to compare and identify any differences. We are also conducting our experiment with neurotypical young adults and young adults in the autism spectrum to investigate potential variations between these groups. Additionally, we are developing computational models that integrate insights from cognitive science and cognitive neuroscience to see if they more accurately match human responses compared to conventional deep learning models.”
Q: What is the impact or value of Rockfish in your research?
LI: “Rockfish is invaluable for our work. It supports everything from preprocessing fMRI data to running complex models and conducting model-brain comparisons. The computing power provided by Rockfish is essential for the success of our projects.”
Learn more about Isik Lab and their work here